Enhancing biotechnological research with Design of Experiments
Biostatistics
- Design of Experiments (DoE) is a powerful statistical tool used to plan, conduct and analyze experiments. It allows to investigate relationships between factors affecting a process and its outcomes while minimizing the number of experiments required.
- Key principles of DoE include randomization (to avoid bias), replication (to increase precision), blocking (to reduce variability), orthogonality, and factorial experimentation (to assess individual and combined factor effects).
- Steps in DoE include defining objectives, identifying key factors and responses, selecting an experimental design, and using statistical analysis to interpret results.
- DoE is particularly useful in biotechnology industry for managing the complexity of biological systems, allowing simultaneous evaluation of multiple factors, which improves understanding, optimization, and quality control in processes.
Background
Are you looking for an effective way to identify which process changes will bring the greatest yield? Do you want to be able to draw valid conclusions from your data with minimal use of resources? If so, you should consider using Design of Experiments (DoE). DoE, also known as experimental design, is a powerful statistical tool used to plan, conduct and analyze experiments in a systematic way. Its primary goal is to explore relationships between various factors that affect a process and outcomes of that process. DoE provides a structured approach to experiments that maximizes the information gained, while minimizing the number of required experiments.1,2
The concept of DoE was introduced in the early 20th century by Sir Ronald Fisher, a British statistician and geneticist. While working at an agricultural research station near London, Fisher recognized the importance of applying statistical analysis not only at the end of experiment, but also during the planning stage. Collaborating with scientists and researchers from various disciplines, he introduced core principles of experimental study design, including randomization, replication and blocking – principles that are still central to today’s practice. His work, in particular the development of the analysis of variance (ANOVA), laid the foundation for modern experimental design, making it an indispensable tool across many scientific disciplines.1,3
In biotechnology, DoE is especially valuable due to the inherent complexity and variability of biological systems. Traditional methods, which change one factor at a time, often miss significant interactions between variables and are time-consuming. DoE solves this problem by enabling simultaneous evaluation of multiple factors and their interactions, providing a deeper understanding of the system under study. In the context of Quality by Design (QbD) approach, understanding both the product and process is crucial to ensuring the quality of the final product. As a result, DoE is a key part of the statistical toolkit used to implement QbD in both research and industrial settings.3-5.
Basic principles of experimental design
The fundamental principles of DoE are designed to improve the efficiency of experiments. These principles include randomization, replication, blocking, orthogonality and factorial experimentation.1,2
Randomization is defined as a process of randomly selecting a sample from a population and randomly selecting the order in which individual experimental runs will be conducted. It helps prevent unknown biases that could distort the results. For example, in a study testing the effect of two different tablets on cholesterol levels, factors like diet and exercise could substantially affect the outcomes. By randomly assigning subject to treatment groups, randomization helps “average out” the influence of such external factors.
Replication is an independent repeat run of each factor combination. This increases both the sample size and precision of the experiment, while allowing to obtain an estimate of the experimental error. Replication reflects sources of variability between and within runs. Although it improves the quality of statistical analysis, it also increases the amount of laboratory work required.
Blocking is a design technique used to reduce or eliminate the impact of known nuisance factors. It works by dividing the study sample into so-called “blocks” – sets of relatively homogenous experimental conditions with low expected variability – which are then separately subjected to the randomization process. For instance, in the above cholesterol study, one of the known nuisance factors might be gender. To counteract its impact, participants can be divided into two blocks, male and female, which are then independently randomized to produce the desired treatment groups. Consequently, the difference between the cholesterol levels induced by the two tablets is not affected by gender. By removing the effect of gender from experimental error, blocking technique increases the precision of the results.
Orthogonality in an experimental design means that the effects of factors are uncorrelated and therefore easier to interpret. In orthogonal designs, factors are varied independently of one another.
Factorial experimentation is used to estimate the effects of individual factors as well as their combinations, by varying them simultaneously and orthogonally. A full factorial design examines all possible combinations of factor levels in each full replication of the experiment. For example, 24 runs are needed to examine four factors, each at two levels (2k factorial design). However, as the number of factors grows, the number of combinations increases exponentially, so fractional factorial designs are often used. These designs require 2k-p runs, where p is the size of the fraction (f. ex. 1 = ½, 2 = ¼ etc.). They still allow to identify the main effects, but without obtaining the complete information on all possible interactions that full factorial designs provide. For example, for five factors at two levels each, full factorial design would need 25 = 32 experiments, whereas fractional factorial design with half fraction chosen would require only 2(5-1) = 16 runs i.e., half as many.
Factorial designs are particularly efficient and widely used in factor screening experiments. They allow for the assessment of between-factor interactions and estimation of factor effects on several levels of other factors, enabling researchers to draw valid conclusions even under complex experimental settings. Owing to their efficiency, fractional factorial designs are often used in studies with four or more factors of interest.
The process of Design of Experiments
When designing an experiment, it is recommended to follow a series of key steps (Figure 1).1
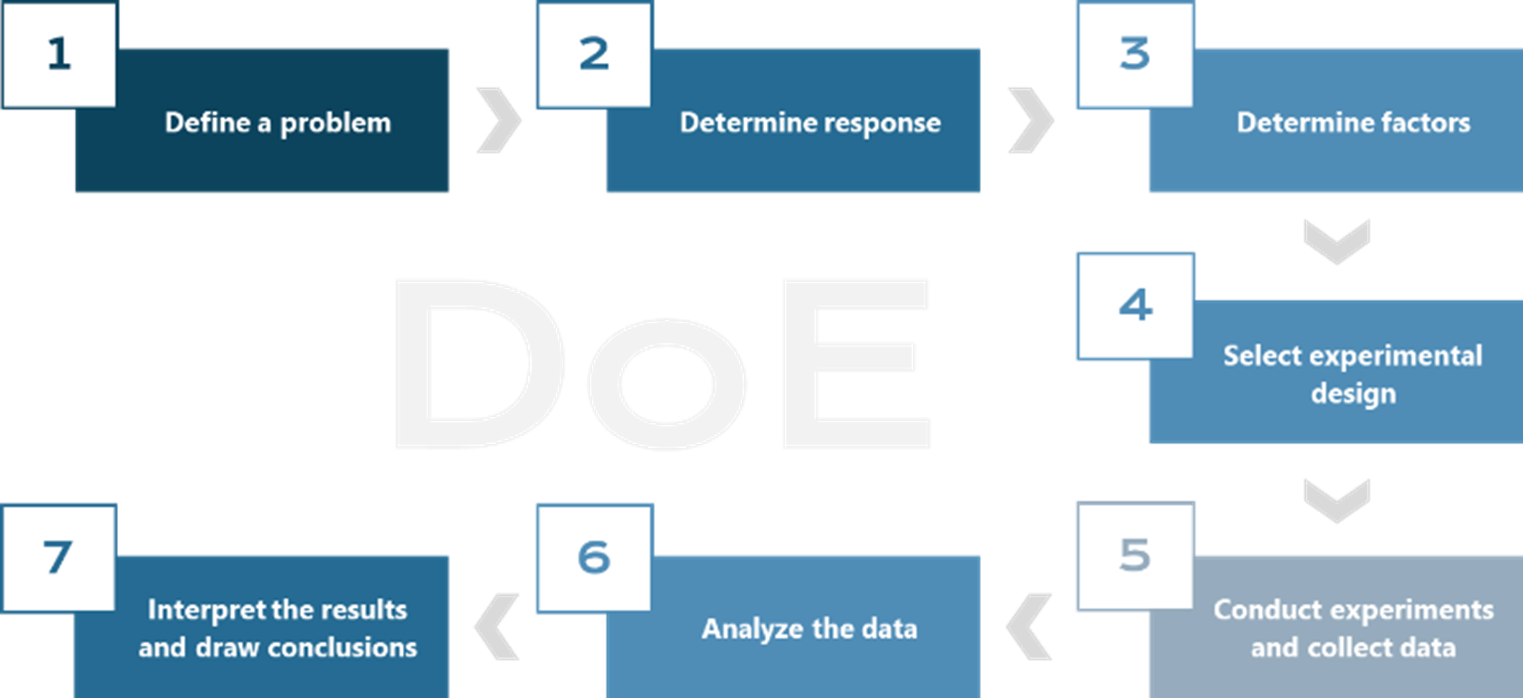
Figure 1 The process of DoE.
- Define a problem
The first step is to clearly define the objective of the experiment. Common objectives include:
- Comparison: Identifying similarities and differences between variables across two or more groups.
- Factor screening or characterization: Identifying the most significant factors that influence the response of interest during the development of a new system or process.
- Optimization: Determining the optimal settings or levels of main factors that yield the desired response values. Typically, an optimization experiment follows a factor screening experiment.
- Confirmation: Ensuring that a system operates or behaves in a way that aligns with a theory or prior experience.
- Discovery: Exploring new materials, factors, or ranges of factors for a given process.
- Robustness: Evaluating how resilient a process is to variations in conditions, and determining how to adjust controlled factors to minimize the impact of uncontrollable ones.
An important part of defining the problem is to understand that a single large, comprehensive experiment may not adequately address all critical questions, especially when knowledge of the studied process is highly limited. A more effective strategy would be to adopt a sequential approach and conduct a series of smaller experiments, each with a focused objective.
- Determine response
Once the objective is established, the next step is to determine the response variable that will provide useful insights into the studied process. A continuous response is generally preferred because it delivers more information than categorical data. It is essential to define how the response will be measured, specify the unit of measurement, and ensure that the measurement system is properly calibrated and maintained throughout the experiment.
- Determine factors
After defining the response, it is necessary to identify the main factors that influence it, the levels of those factors, and their ranges. It is also essential to consider nuisance factors that might affect the response but are not of interest. As mentioned earlier, blocking and randomization are effective ways to manage these nuisance factors.
- Select experimental design
When choosing an experimental design, it is crucial to consider the sample size (number of replicates), the run order for experimental trials, and whether blocking is necessary. A variety of statistical software can assist with this process, allowing experimenters to input factors, levels and ranges, and offering design recommendations. Most of these programs also provide performance diagnostics to help evaluate design alternatives and generate a randomized worksheet for the experiment.
- Conduct experiments and collect data
After defining all variables and selecting the appropriate experimental design, one can proceed to the actual experiment. The experiment must be conducted strictly according to the predetermined design plan. Errors or deviations from the experimental procedure usually compromise the validity of the study.
- Analyze the data
The gathered data should be analyzed with appropriate statistical methods, which often involve ANOVA and various graphical techniques. The approach to data analysis depends directly on the chosen design.
- Interpret the results and draw conclusions
Finally, the obtained results must be presented and interpreted both statistically and in relation to existing theories, to deepen the understanding of the process. The evaluation of the DoE results can help make scientifically grounded decisions about the next steps, such as conducting confirmatory trials, augmenting designs, or advancing to scale-up and technology transfer.1-4
Applications of Design of Experiments
DoE is used in many branches of biotechnological and pharmaceutical industries, which include, but are not limited to: analytical method study and development6,7, pharmaceutical processes8,9, drug delivery10, drug formulation11, biomanufacturing optimization12 and bioanalytical methods.13
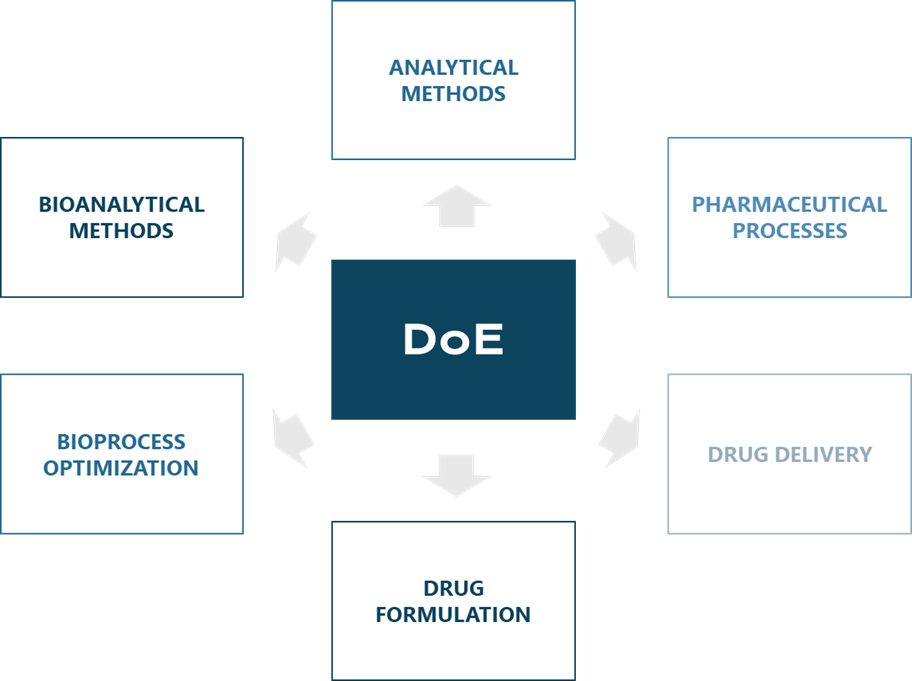
Figure 2 Various applications of DoE.
Case study
At Mabion, we have successfully applied DoE methods in many projects including the process characterisation study (PCS) for bioreactor cell culture in the protein production process. The primary objective of the study was to define Proven Acceptance Ranges (PARs) and Normal Operating Ranges (NORs) for critical process parameters, and to establish acceptance criteria for these parameters. The experiment was designed based on data from the preliminary OFAT (One-Factor-At-a-Time) experiment. Two DOE studies were conducted as a part of the PCS: DOE1 (screening study) and DOE2 (for final process parameter classification).
DOE1 employed a 5-parameter fractional factorial design that investigated parameters such as seeding density, temperature, pH, cell culture duration and oxygenation (Table 1). These parameters were evaluated against 11 different response variables, classified as Process Performance Attributes (PPAs) or Quality Product Attributes / Critical Quality Product Attributes (QPAs/CQPAs). The analysis of DoE1 data led to the classification of cell culture duration as Key Process Parameter (KPP) and oxygenation as Critical Process Parameter (CPP) with corresponding NORs and PARs defined for both.
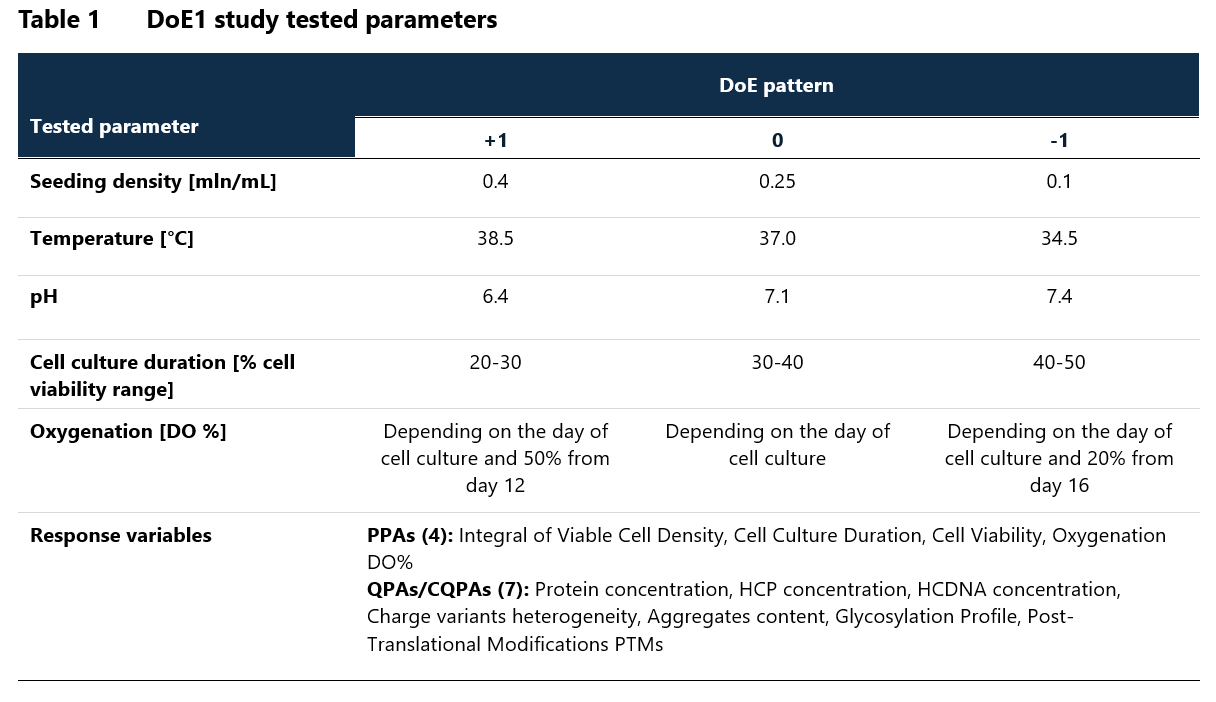
Based on the results of the DoE1 study, DoE2 study employing a 3-parameter full factorial design was designed, focusing on evaluation of seeding density, temperature and pH (Table 2). The parameter levels were refined according to DOE1 results while the response variables remained the same. The initial classification of tested parameters was re-evaluated on the basis of obtained results and statistical analysis.
Based on DoE2, temperature and pH were classified as CPPs, while seeding density remained as KPP. NORs and PARs were defined for all tested parameters.
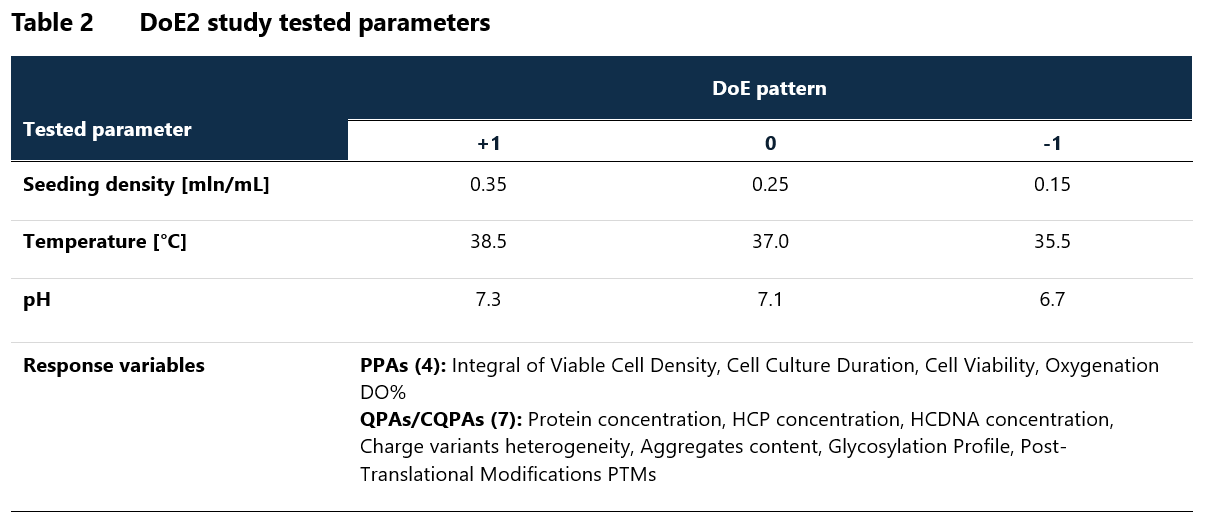
The application of DoE in the process characterization study provided us with a deeper understanding of critical process parameters and their influence on product quality. This approach has also helped to establish a design space that ensures optimal process performance, thereby improving efficiency.
In summary, the use of DoE approach in biotechnological industry offers several advantages:
- Accelerates development
Reduces the time required for production or method development, leading to faster results.
- Improves product quality
Enhances product consistency and ensures that the final product meets high standards.
- Boosts formulation stability
Increases the stability and efficacy of new formulations, ensuring product safety and effectiveness.
- Supports regulatory compliance
Helps meet industry standards and regulatory requirements.
- Controls costs
Plays a significant role in managing production and development costs.
- Optimizes resource use
Ensures efficient utilization of resources such as equipment, electricity and water, leading to significant savings.
Conclusions
A properly designed experiment offers substantial benefits, increasing both the productivity and research precision. By applying Design of Experiments (DoE), you can maximize the efficiency of your processes while ensuring accurate and reliable results. DoE allows you to explore multiple factors simultaneously, identify critical variables, and understand their interactions—leading to better decision-making and more robust conclusions.
To fully leverage the advantages of DoE, it is important to adhere to its core principles—randomization, replication, and blocking—and follow the essential steps in designing an experiment. Begin by clearly defining the objective of your experiment, whether it’s for comparison, optimization, or screening of factors. Carefully select your response variables and main factors, paying attention to nuisance factors that may influence your results. Use statistical software to assist in generating an optimal design and ensure the experiment is executed exactly as planned. Proper analysis of your results, often involving ANOVA and graphical methods, will allow you to draw valid conclusions and refine your process.
Incorporating DoE into your research and development workflows will not only save time and resources but also improve the quality and consistency of your outputs. It’s a powerful tool for process optimization, cost reduction, and meeting regulatory standards, making it an invaluable asset in today’s competitive and data-driven landscape.
Prepared by:
Urszula Wójcik-Bojek
References
- Montgomery, D.C. (2013) Design and analysis of experiments. 8th ed. New York: John Wiley Sons, Inc. ISBN 9781118214718.
- Telford, J. (2007). A brief introduction to design of experiments. Johns Hopkins Apl Technical Digest, 27, 224–232.
- N Politis, S., Colombo, P., Colombo, G. et al. (2017). Design of experiments (DoE) in pharmaceutical development. Drug development and industrial pharmacy, 43, 889–901.
- Dhoot, A.S., Fernandes, G.J., Naha, A. et al. (2019). Design of experiments in pharmaceutical development. Pharm Chem J, 53, 730–735.
- ICH Q8 (R2) Pharmaceutical Development, Guidelines (2009). Available at: https://www.ema.europa.eu/en/ich-q8-r2-pharmaceutical-development-scientific-guideline. (Accessed August 28, 2024).
- Little, T.A. (2014). Design of experiments for analytical method development and validation. BioPharm International, 27, 40–45.
- Sakr, M., Hanafi, R., Fouad, M., Al-Easa, H., El-Moghazy, S. (2019). Design and optimization of a luminescent Samarium complex of isoprenaline: A chemometric approach based on Factorial design and Box-Behnken response surface methodology. Spectrochimica acta. Part A, Molecular and biomolecular spectroscopy, 208, 114–123.
- Korhonen, K., Poikolainen, M., Korhonen, O., et al. (2016). Systematic evaluation of a spraying method for preparing thin Eudragit-drug films by Design of Experiments. J Drug Deliv Sci Technol, 35, 241–251.
- Nair, A., Khunt, D., Misra, M. (2019). Application of quality by design for optimization of spray drying process used in drying of Risperidone nanosuspension. Powder Technol, 342, 156–165.
- Kumar, P. M., Ghosh, A. (2017). Development and evaluation of silver sulfadiazine loaded microsponge based gel for partial thickness (second degree) burn wounds. Eur J Pharm Sci, 96, 243–254.
- Hwang, R-ch., Kowalski, D.L. Design of Experiments for Formulation Development https://www.pharmtech.com/view/design-experiments-formulation-development-0 (Accessed September 12, 2024).
- Kasemiire, A., Avohou, H.T., De Bleye, C., et al. (2021). Design of experiments and design space approaches in the pharmaceutical bioprocess optimization. Eur J Pharm Biopharm, 166, 144-154.
- Reddy, M. S., Kumar, I. , Attari, Z. et al. (2017). Statistical Optimization of Extraction Process for the Quantification of Valsartan in Rabbit Plasma by a HPLC Method. Indian Pharm Sci, 79, 16–28.